
There a bunch of reasons why videos look awful and bad, and blurry, and unpleasant to view.
The most common reason for blurry videos is that they were recorded or encoded at a low resolution. Then, if there is too much compression is used, it will also degrade quality. In all, low quality videos are the result of the elimination of details that are irreversible.
That, before AI takes the seat.
A team of video and AI engineers at Adobe Research has developed an AI application called 'VideoGigaGAN'.
What it does, is enhancing blurry videos, sharpening them to 8×.
Unlike other Video Super Resolution (VSR) tools which can also enhance low-quality videos, Adobe said that VideoGigaGAN's superiority is due to its way of enhancing videos by avoiding strange AI artifacts.
This way, results should have more fine-grained details.

In a more detailed explanation, Adobe said that:
"We also enhance consistency by incorporating the features from the flow-guided propagation module."
"To suppress aliasing artifacts, we use Anti-aliasing block in the downsampling layers of the encoder."
"Lastly, we directly shuttle the high frequency features via skip connection to the decoder layers to compensate for the loss of details in the BlurPool process."
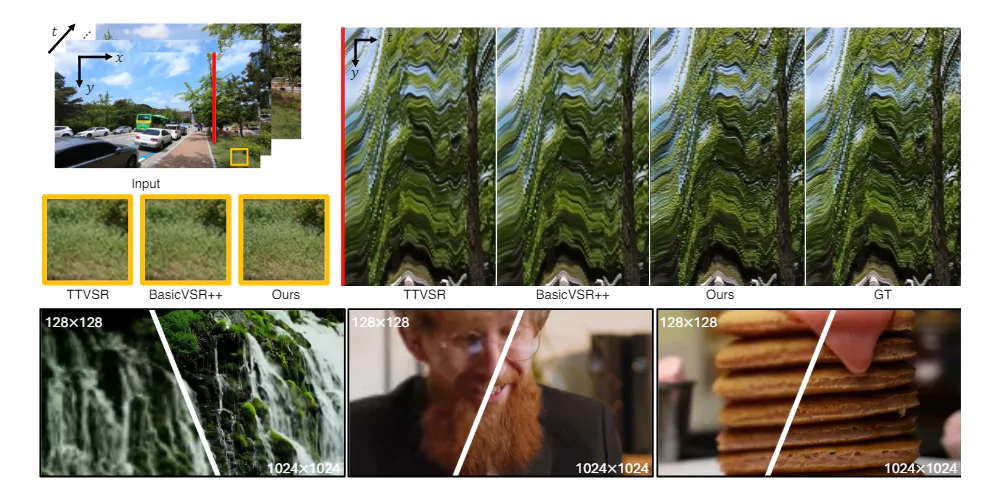
Another way of saying this, the AI 'imagines' what the image in each frame should look like, and recreate it.
AI weirdness is suppressed to a very minimum, and this makes VideoGigaGAN different than other VSRs.
The team used what's called an 'Adam optimizer' for training with a fixed learning rate of 5 × 10−5. Then, during the training, the team randomly cropped a 64 × 64 patch from each LR input frames at the same location, and used 10 frames of each video and a batch size of 32.
The batch is distributed into 32 Nvidia A100 GPUs.
The total number of training iterations for each model is 100,000.
Built upon the large-scale upscaler GigaGAN, it improves the temporal consistency of unsampled videos by adding temporal modules and enhancing techniques.
AI applications have been in the news a lot lately, primarily due to the release of LLMs, such as OpenAI's ChatGPT, that users can use to generate a wide variety of output.
But AI research has been ongoing in other areas as well, such as creating artificial images and video.
VideoGigaGAN is not a generative AI, in which it doesn't response to prompts and queries.
Instead, it imagines existing material, and build information based on that.
This makes it unlike OpenAI Sora, for example.
The team at Adobe described their work and results in an article (PDF) posted to the arXiv preprint server.
They have also posted several examples of the videos that they have enhanced on their project website page.