Humans are still dependent on fossil fuels that are finite in amount. This is why everything we do that involves the processing and the using of fossil fuels should be carefully calculated
When AI starts getting traction, the industry often compared to the oil industry, where once refined, the product can become a highly lucrative commodity. This goes further, as people starts to realize the environmental impact of such technology.
In a paper, researchers at the University of Massachusetts performed a life cycle assessment for training several common large AI models.
Here, they found that the process can emit more than 626,000 pounds of carbon dioxide.
To put it in another perspective, this is an equivalent of nearly five times the lifetime emissions of the average car.
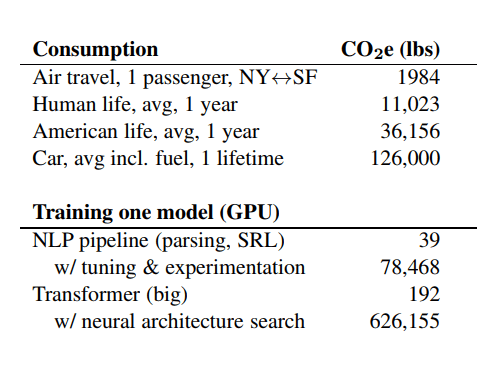
According to Carlos Gómez-Rodríguez, a computer scientist at the University of A Coruña in Spain, who was not involved in the research:
"Neither I nor other researchers I’ve discussed them with thought the environmental impact was that substantial."
In the paper, the researchers specifically examined the model training process for natural-language processing (NLP), the subfield of AI that focuses on teaching machines to handle human language.
Since 2017, the NLP community has reached several noteworthy performance milestones in machine learning technology, but such achievements have required training AI models that were increasingly larger.
And this approach is computationally expensive, and not to mention, highly energy intensive.
The researchers looked at four models in the field that have been responsible for the biggest leaps in performance: the Transformer, ELMo, BERT, and GPT-2.
Here, the researchers trained each of the model on a single GPU for up to a day to measure its power usage. With the data, the researchers then used the number of training hours listed on the model's original paper to calculate how much energy each of the model consumed to complete its training process.
That number was converted into pounds of carbon dioxide equivalent, which is close to the energy mix used by Amazon’s AWS, the largest cloud services provider.
Making things worse, the researchers also found that the computational and environmental costs of training grew proportionally to the model size. The costs can even escalate much higher when the AI model's training involves additional tuning steps to increase its final accuracy.
In particular, the researchers found that a tuning process known as neural architecture search, which aims to optimize a model by incrementally tweaking a neural network’s design. This process is known to be quite exhaustive in trials and errors, and the researchers found that it had extraordinarily high associated costs for just a small performance benefit.
Without it, the most costly model, BERT, had a carbon footprint of roughly 1,400 pounds of carbon dioxide equivalent. This is around the same as a the environmental cost for a round-trip trans-America flight for one person.
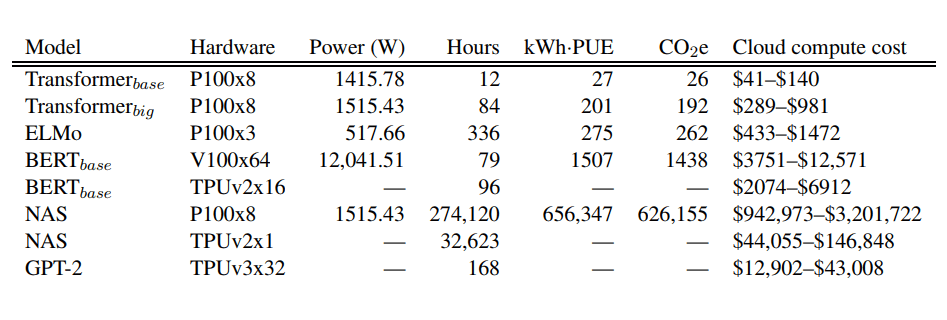
The researchers noted that the figures should only be considered as baselines.
"Training a single model is the minimum amount of work you can do,” says Emma Strubell, a PhD candidate at the University of Massachusetts, Amherst, and the lead author of the paper.
What this means in practice, it’s much more likely that AI researchers would develop a new model from scratch or adapt an existing model with a new data set. Which in either way, can require many more rounds of training and tuning.
The significance of those figures is colossal, especially when considering the trends in AI research which are getting more intensive.
Since humans are still reliant on fossil fuels, the increasing usage of AI should spark some environmental concerns.
This is because fossil fuels, which are formed by natural processes, such as anaerobic decomposition of buried dead organisms, containing energy originating in ancient photosynthesis, typically requires millions of to form.
The world's primary energy sources consists of petroleum, coal, and natural gas, and these resources are depleting rapidly.