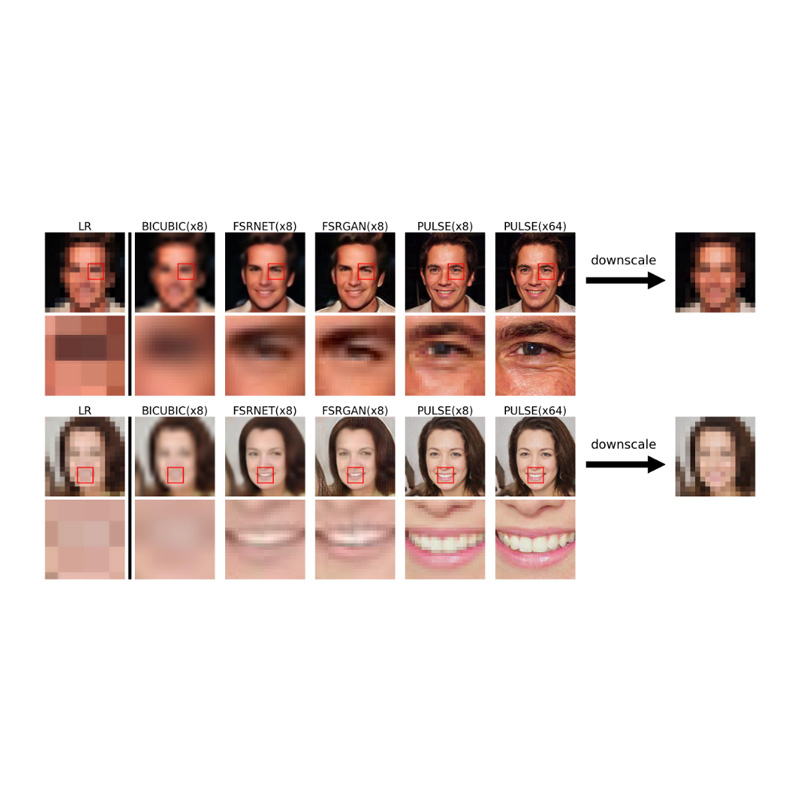
In the modern days of technology, almost everyone in the world have smartphones. With those devices, people can capture almost anything as soon as it happens.
However, even as digital cameras have gotten better in each coming generation of the technology, the best photographers can still get the occasional unwanted blurry shots.
From malfunctioning software, glass/lens issues, sudden movement, incorrect shutter speed, issues in focus, poor lighting, camera specs, and more, blurs in images can be caused by a lot of factors,
For that reason, no person can take perfect photos every time.
Blurry images won't go away anytime soon. Not only people need to work with their ability in taking photos, as technology companies are also required to ramp up their efforts in improving the technology.
There is a workaround though.
Using AI, smartphone vendors have tried to eliminate blurs to some degree. But none has gone as far as this 'PULSE' AI tool from the researchers at Duke University.
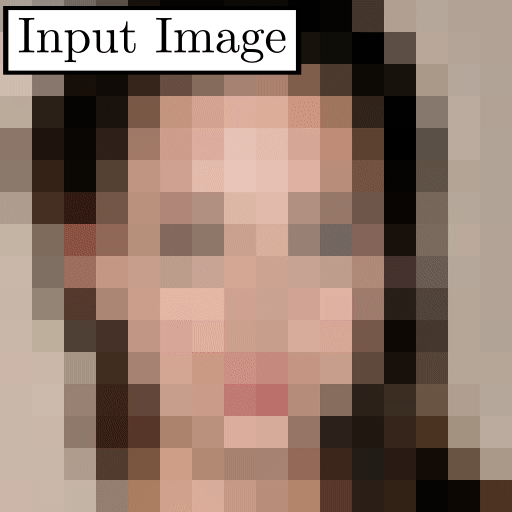
The researchers use a novel approach to photo correction, by making the AI work by searching through its learnt AI-generated images of high-quality faces, to find the ones that look like the input image, to the compress the found images to the same size as the input.
The AI-generated photos were created using generative adversarial networks (GANs), which produce new images by pitting two neural networks against each other. The first agent of the two generates new images and the second agent checks whether how they look.
Success is when the first network gets better with experience until the second thinks the images could pass as the real thing.
The team said that PULSE can turn a handful of pixels into realistic faces with up to 64 times the resolution of the input. The researchers claimed that the AI can even “imagine” new human traits and features that were imperceptible in the original, such as wrinkles and eyelashes.
On top of that, the researchers also think that the AI could create pictures for a range of other applications, from microscopy to satellite imagery.
“Never have super-resolution images been created at this resolution before with this much detail,” said Duke computer scientist Cynthia Rudin, who led the research team.
From the researchers' web page:
"In previous approaches, which have generally been supervised, the training objective typically measures a pixel-wise average distance between the super-resolved (SR) and HR images. Optimizing such metrics often leads to blurring, especially in high variance (detailed) regions."
"We propose an alternative formulation of the super-resolution problem based on creating realistic SR images that downscale correctly."
"We present a novel super-resolution algorithm addressing this problem, PULSE (Photo Upsampling via Latent Space Exploration), which generates high-resolution, realistic images at resolutions previously unseen in the literature. It accomplishes this in an entirely self-supervised fashion and is not confined to a specific degradation operator used during training, unlike previous methods (which require training on databases of LR-HR image pairs for supervised learning)."
"Instead of starting with the LR image and slowly adding detail, PULSE traverses the high-resolution natural image manifold, searching for images that downscale to the original LR image. This is formalized through the “downscaling loss,” which guides exploration through the latent space of a generative model."
"By leveraging properties of high-dimensional Gaussians, we restrict the search space to guarantee that our outputs are realistic. PULSE thereby generates super-resolved images that both are realistic and downscale correctly."
Adding that the state-of-the-art methods in perceptual quality can create higher resolutions and scale factors than previously possible, the researchers said that the project is based on Dmitry Ulyanov's Deep Image Prior project page.
Deep Image Prior uses "a randomly-initialized neural network" that "can be used as a handcrafted prior with excellent results in standard inverse problems such as denoising, super-resolution, and inpainting."
Usually, deep convolutional networks are popular tools for image generation and restoration. Generally, their excellent performance is imputed to their ability to learn realistic image priors from a large number of example images.
But Deep Image Prior, and Pulse in this case, have their generator network capable of capturing a great deal of low-level image statistics prior to any learning.
Funded by the Lord Foundation of North Carolina and the Duke Department of Computer Science, the novel methodology for image bridges the gap between two very popular families of image restoration methods: learning-based methods using deep convolutional networks and learning-free methods based on handcrafted image priors such as self-similarity.
What makes it a potential future for improving existing technology, according to the researchers on their paper, is because it had no problems when applied to different domains.
The researchers have made the project open sourced on GitHub.