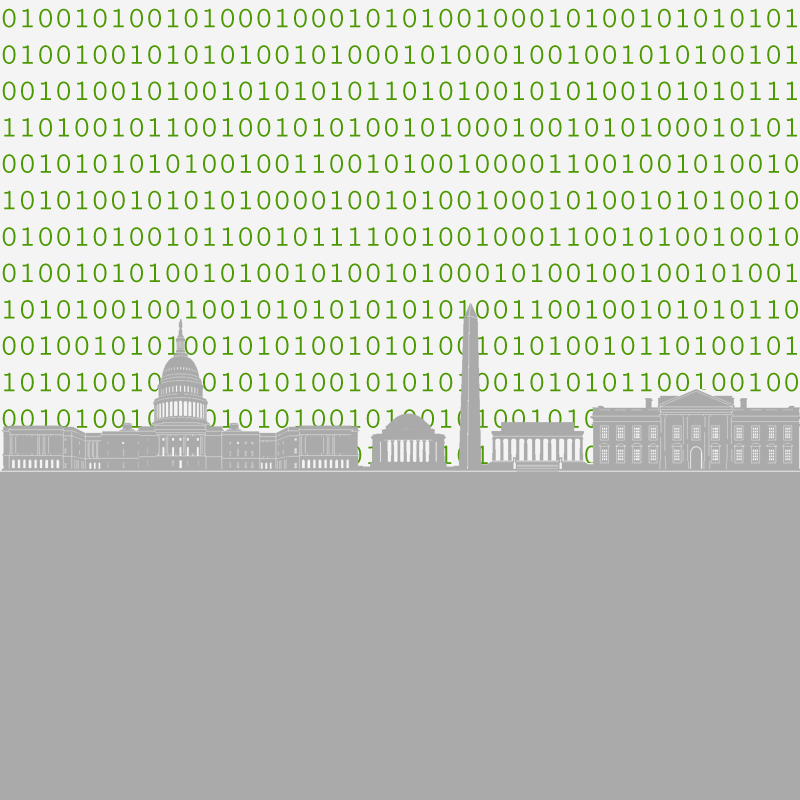
Not only companies that want to use AI to benefit their business, as governments too want to leverage the technology for specific goals.
With AI becoming common, and powering many parts of automation, calculation, research and more, the U.S. Department of Defense has put a particular interest in AI.
Here, the Defense Advanced Research Projects Agency (DARPA) is pursuing an unprecedented machine-learning "breakthrough" technology.
This includes pioneering a cybersecurity method intended to thwart multiple attacks at once, and stop newer attacks from becoming less recognizable to existing defenses.
The fundamental of the project, is to use machine learning technology to make systems better in identifying, integrating and organizing future information that can be complicated, sophisticated or never yet encountered.
Using what's called the 'Lifelong Learning Machines' (L2M), DARPA intends to massively improve real-time AI and machine learning technology using a cybersecurity concept called 'GARD', or 'Guaranteeing AI Robustness Against Deception' (PDF).

"If something new is different enough, the system may fail. This is why I wanted to have some kind of machine learning that learns during experiences. Systems do not know what to do in some situations," said Dr. Hava Siegelmann, program manager in DARPA's Information Innovation Office (I2O) and Professor of Computer Science at the University of Massachusetts.
In the modern era where information thrive, new things can come up.
Security systems which are usually based and created by past references, may struggle to understand and recognize new attacks that never yet encountered.
“Current defense efforts were designed to protect against specific, pre-defined adversarial attacks and, remained vulnerable to attacks outside their design parameters when tested. GARD seeks to approach machine learning defense differently,” the DARPA official explained.
Using machine learning, the goal could be explained in terms of immediate "real-time training."
If machines learn even the most difficult things while performing analysis in real time, then, according to Siegelmann, "we are not bound to the training set (previously compiled or stored information). We Put old data and new data all together to retrain the network on all the training data."
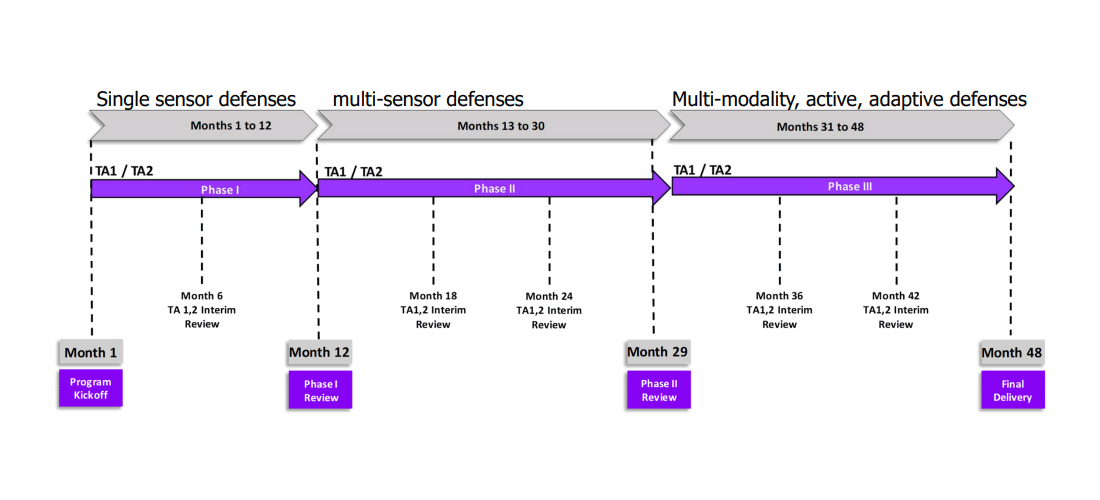
"Over the last decade, researchers have focused on realizing practical ML capable of accomplishing real-world tasks and making them more efficient," continued Siegelmann.
"We're already benefiting from that work, and rapidly incorporating ML into a number of enterprises. But, in a very real way, we've rushed ahead, paying little attention to vulnerabilities inherent in ML platforms – particularly in terms of altering, corrupting or deceiving these systems."
While using AI to make systems more capable is certainly an advantage, there are some disadvantages too.
Siegelmann explained that there are certain kinds of never-before-seen nuances or data permutations a machine-learning can typically analyze.
For example, the technology may not yet have the ability to fully understand, digest and assimilate some very subjective variables such as “feelings” or ”instincts”, or other kinds of nuanced decision-making uniquely enabled by human cognition.
There are also things that are not compatible with computer algorithms, mathematical formulas or some purely scientific methods of analysis.
However, AIs can be trained with a lot of data, and in theory, the more it learns the better it can be.
Since the modern era of technology has given the world an increasing amount of information, the AI can learn from this massive trove. This should make the system capable of drawing conclusion from databases that include things like speech patterns, prior behavior and other kinds of cataloged evidence.
This is why AI is a field considered to be the cutting edge technology capable of handling data, including those that are more subjective phenomena.
"When a baby is born it is learning all the time to adapt and learn all the time. People are afraid of surprises. This is precisely the point; the faster a machine is able to absorb and process new information by instantly adding it and synchronizing with its existing database, the faster it can train to recognize and compute new things," she said.
"We will be making AI better to create defenses so existing machine learning will be defendable, by either defending the current one or making new machine learning," added Siegelmann.