In the past, Hollywood can spend millions of dollars on computers, talents and special effects to make up a realistic scene.
With those at their disposal, whatever is on screen, should never be taken for granted. But those days are gone.
With artificial intelligence and cloud computing, even amateurs with just a few hundred dollars and some time to spend could create something that is almost as true to life.
Deepfake that started out from a user on Reddit, makes it possible for anyone even with a little knowledge of computing, to put someone's face, superimposing it on another person's body.
This way, the technology can create videos and scenes to include a person that was never in them.
The technology is certainly entertain. But unfortunately, the technology can, and has been for many times, used for things that are sinister.
With deepfakes, people can create pornography without the consent of the people depicted. In fact, most deepfakes are porn related.
People can also make high-profile influential figures and politicians to say something they never said.
And worse, they can also use deepfakes to create doubt of real videos, by suggesting that the real ones are deepfakes.
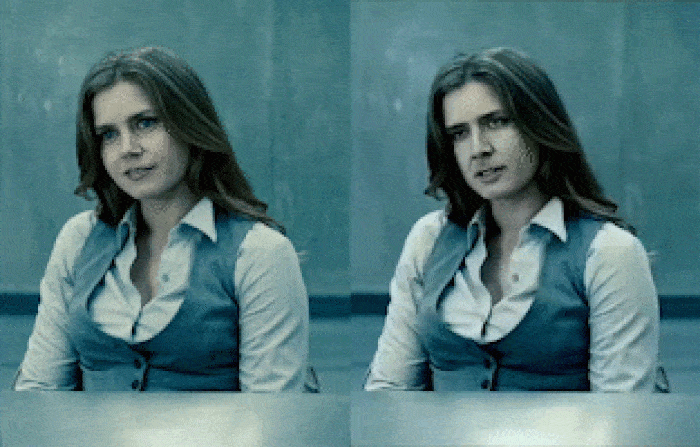
Most people know that they shouldn't believe everything they see on screens. Photoshopped images are the first that comes in mind when thinking about manipulation, for example.
But deepfakes go far beyond Photoshopping. The technology can manipulate videos to various degrees that some can find it eerily scary.
Understanding the risks, there should be tools present that can detect deepfakes and label them clearly.
These tools are called deepfake detectors. And when they are present, the war begins.
As researchers know more about how this technology works, and develop the so-called deepfake detectors, we could see many uses for these tools in the future.
Deepfake detectors would help ensure that fake videos won't spread misinformation, disinformation or malinformation to fool the public, and at the same time allow real videos to be received as authentic and legit.
Journalists can use this kind of tools to verify information before publishing it. They can then warn people about the deepfakes whenever they are detected. Social media networks can use the tool to create digital fingerprints of the deepfakes, so they cannot be reshared.
These tools however, won’t solve all deepfake problems. They are just one part of the arsenal in the broader fight against disinformation.
This is because deepfake detection tools came not long after deepfakes started to plague the web. Most notably, after actress Gal Gadot had her face superimposed to a porn star's body.
Early works focused on detecting visible problems in the videos, such as deepfakes that didn’t blink.
With time, however, deepfakes have gotten so much better at mimicking real videos and become harder to spot for detection tools.
But again, researchers and tech companies are investing heavily on creating better detection tools.
With their resources, they can, for example, train AIs to learn from patterns from individuals that have a lot of videos circulating online, to understand their body languages. By understanding how the person stands or do hand gestures, detectors can possibly detect deepfakes, even when the quality of the video is bad.
Then there is a way to extract essential data from frames, and track them through sets of concurrent frames to detect any inconsistencies.
There are also techniques that use blockchain to verify the source of the media through the ledger. This way, only videos from trusted sources would be approved, decreasing the spread of possibly deepfake media.
And again, the technology behind deepfake improves.
It's an AI race for supremacy; to see which is one is better.
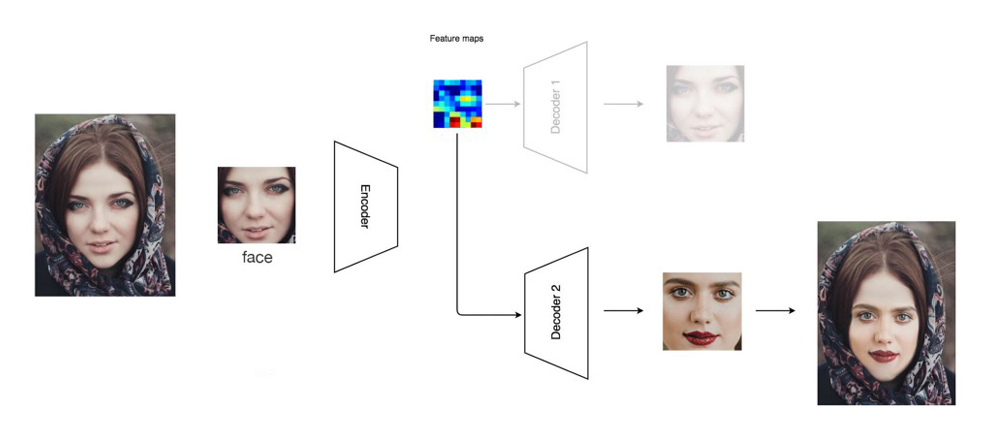
But no matter how good deepfake detectors can be, they are useless if people don't see deepfakes as something damaging, and keep on spreading the fakes despite knowing that they are fakes.
Part of the problem with automating the detection of deepfakes, is that some people see certain deepfakes as entertaining while others see them as harmful. In other words, as will other forms of hoaxes, without context, the tools can be useless.
In this case, a deepfake label won't be enough.
Then there is the cat and mouse game, where a detector algorithm can be trained to spot deepfakes, but then an algorithm that generates deepfakes can potentially be trained to evade detection.
Next, is performance. No detection tool can be perfect. Even if it has a detection rate of 99% accuracy, for example, the remaining 1% could still be damaging when thinking at the scale of internet platforms.
And for last, the internet provides frictionless sharing, with many people are seeking attention as their base for monetization. What this means, deepfakes will always find an audience.
So here, creating a really useful deepfake detector can be even harder than what was previously thought.
Because deepfakes are here to stay, managing disinformation and protecting the public will be much more challenging than ever as AI gets more powerful.
Humans should take part by acknowledging how harmful deepfakes are posing to the society, in the hands of malicious actors. We, are all part of a growing research community that should take this threat's challenge. And detection tool, is just the first.
Further reading: How To Easily And Quickly Spot Deepfake Whenever You See One