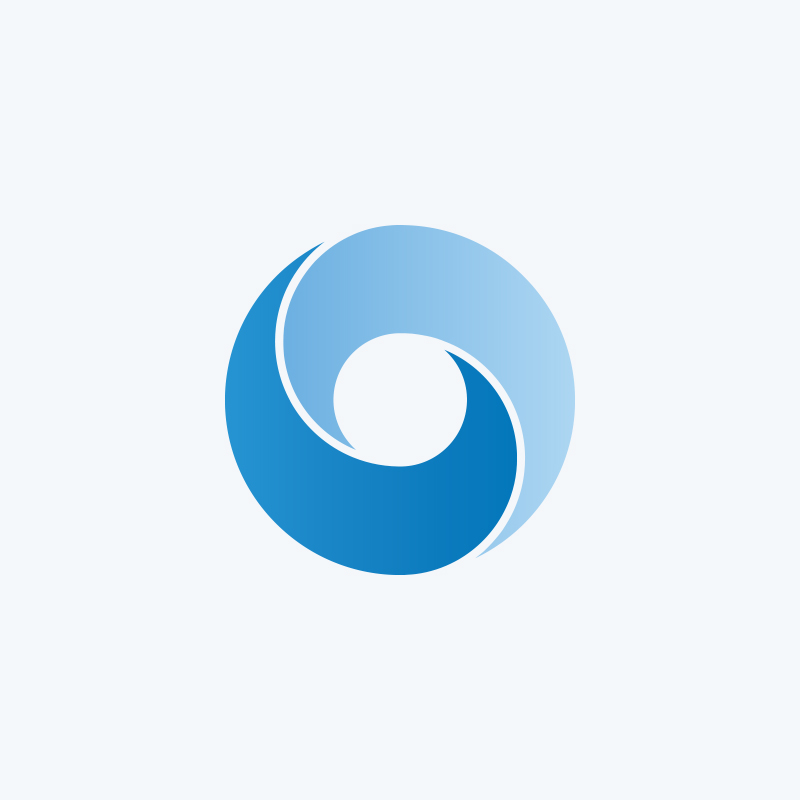
Humans will do whatever they can in order to survive. We know that helping each other has an advantage to overcome many situations. But we also know that in some circumstances, betrayal can be the key for survival. The same goes for machines.
Technology companies have been working to improve their research and application on Artificial Intelligence (AI), and enhancing how they can aid humans in many tasks, including the Internet of Things, computing and many others. They are also continuously enhanced to ensure safety and security in various fields.
Here AI needs to have the ability to overcome situations where complicated problems happen. To make this happens, machines need to learn beyond the boundaries of their algorithms, they need to understand the world they see and work with other technological agents when needed.
This is where Google's DeepMind researchers do their research.
After creating AI that was able to defeat a human professional Go player, the researchers are on to another attempt in understand the capabilities of AI. On their research, they pitted AI agents with each other in two game simulations to determine how they behave in certain circumstances under certain rules.
The simulations were meant to extent the cooperation between different AIs when thrown in a social dilemma, by recreating tests similar to the "Prisoner's Dilemma".
And after the two AIs played 40 million different turns, the results are interesting.
When Cooperation is the Solution to Survive
The first scenario, shown above, is called the Wolfpack simulation.
Here, the AIs are rewarded for working together. The two AIs are made as hunters (red color), going against another AI that poses as a prey (blue color). The hunter AIs stalk and capture the prey around the board. While they can certainly do this individually, but the lone wolf can lose the carcass to scavengers.
The two AIs see the rules and understand that working together is the best to overcome the problem. The AI would sometimes seek each other and search together. On other times, one would spot the prey and wait while the other hunts.
As the benefit of cooperation was increased by researchers, they found the rate of lone-wolf captures went down dramatically.
Survival of the Fittest
The second scenario is the Gathering simulation, a different one from the Wolfpack hunting game. In the simulation, the two AIs (indicated by red and blue squares) move across the grid in order to pick up green "fruit" squares. Each time the player picks up fruit, it gets a point and the green square goes away. The fruit respawns after some time.
The AIs are given the option to fire a laser beam to the opponent AI. But when the fruits are plenty, they tend to play peacefully. They learn that shooting the opponent takes time and precision so the AI simply ignores shooting in favor of gathering more fruits.
The AI works individually to collect each fruits by playing a fair game. But when DeepMind's researchers modified the respawn rate of the fruits, they noted that the desire to eliminate the other player emerges "quite early." Here, they started to become aggressive.
The two AI agents know that hitting the opponent will remove it from the game for several frames, giving it an advantage over the opponent in gathering more fruits.
The AIs at first coexist peacefully. But when scarcity is introduced, they started 'killing' each other by shooting beams.
Conclusion
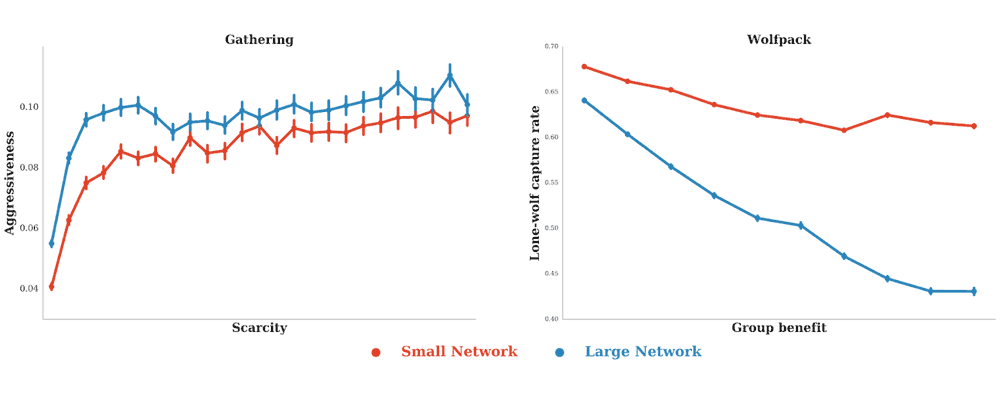
DeepMind said that these simulations illustrate the concept of temporal discounting. When a reward is too distant, people tend to disregard it. This is somehow the same for neural networks.
In the first scenario, resources are abundance. The two AIs see cooperation as something beneficial, so the two work together. But when resources are scarce, the machines start behaving aggressive, in a human-like manner.
In the Gathering simulation, shooting the other AI player delays the reward slightly, but it give it a chance to gather more fruit without competition. But in the Wolfpack scenario, the AI learned that acting alone is more dangerous. Here they do the opposite by delaying the reward in order to cooperate.
What is worth noting is that in the Wolfpack simulation, the researchers said that the smarter the AI, the more it cooperates in order to corner and capture the prey. However, in the Gathering simulation, the more complex the AI's deep learning system is, the more aggressive it becomes. The researchers noted that, since the process of shooting requires complex strategies, smarter AIs tend to be more aggressive and shoot its competition earlier and more frequently to get ahead of the game regardless of the fruit supply.
And what made it interesting is that the two AIs were able to adapt to situations and modify their behavior accordingly to be victorious.
While humans have the tendencies to distrust AI based on the negative impressions left by many science fiction stories, DeepMind is showing that there are indeed warnings and complications that can happen when technology improves.
"Our experiments show how conflict can emerge from competition over shared resources and shed light on how the sequential nature of real world social dilemmas affects cooperation."
However, the benefit for AI to work alongside humans is huge. "[Such] models give us the unique ability to test policies and interventions into simulated systems of interacting agents - both human and artificial," said the team.
DeepMind suggests that neural network learning can provide new insights into classic social science concepts. It could be used to test policies and interventions with what economists would call a "rational agent model." This may have applications in economics, health to environmental science and many others.
Related: Scientists Are Teaching Artificial Intelligence To Kill Each Other In A Deathmatch