
With the available tools, bad actors are getting better in generating fake news and misinformation to fool readers into thinking that they are legitimate.
Using AI-based text generators for example, including the OpenAI's GPT-2, computers have helped those bad actors with their intentions.
To mitigate this, researchers have started fighting AI-based fake news with AI-based fake news detector, which work by essentially tracing back a text writing style to determine if it was written by humans or bots.
However, these kind of AI-based fake news detectors assume that text written by humans is always legitimate and the text generated by bots is always fake. In other words, even if an AI is capable of generating legitimate text, the fake news detector will mark it as a fake.
According to researchers at MIT’s Computer Science and Artificial Intelligence Laboratory (CSAIL), AI-based fake news detectors like the GLTR, could be fooled with factually true articles because a fundamental flaw may be present in its AI detection method.
Their finding is based on the nature of AIs, that hunger for data to learn for patterns.
The team also suggest that bad actors can also use the tools to manipulate human-generated text. The team at MIT realized this after training an AI using the GPT-2 model, and saw how the results can be corrupted to alter their meaning.
Tal Schuster, an MIT student and lead author on the research, said that it’s important to detect factual falseness of a text rather than determining if it was generated by a machine or a human:
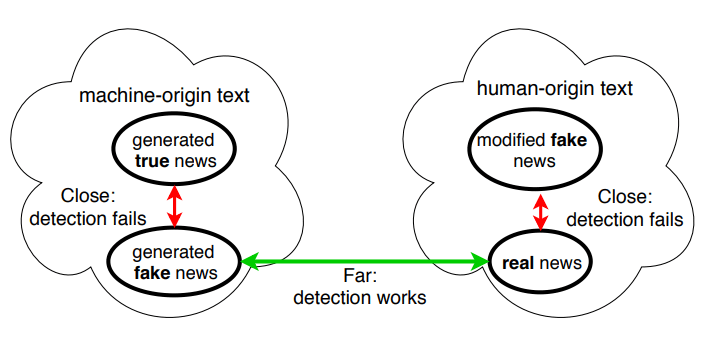
MIT professor Regina Barzilay said this research highlighted the lack of credibility of misinformation classifiers.
To overcome these flaws, the researchers used Fact Extraction and VERification (FEVER), the world's largest fact-checking database with evidence from Wikipedia articles, to develop the next-generation new detection systems.
However, the research team found the model developed through FEVER was prone to errors due to the datasets’ bias. Negated phrases for example, are often deemed to be false by the model, said Schuster.
This happened because many of the data inside the datasets were created by human annotators, which may contain give-away phrases. In this case, phrases like "did not" and "yet to" appear mostly in false statements.
And when the team de-biased FEVER, the detection model's accuracy fell from 86% to 58%.
The model didn't perform well on the unbiased evaluation sets, because the researchers chalked up to those models’ overreliance on the bias to which they were initially exposed to. Schuster said that the cause was the model in taking into account languages without any external evidence.
Schuster suggested that a detector can deem a future event as false, just because it hasn't used external sources as part of its verification process.
The final fix involved engineering an entirely new algorithm, which is trained on the de-biased dataset, by combining both fact-checking and existing defense mechanisms.
The work is far from over, and the team is hoping that future models can use this approach to better detect new types of fake news.