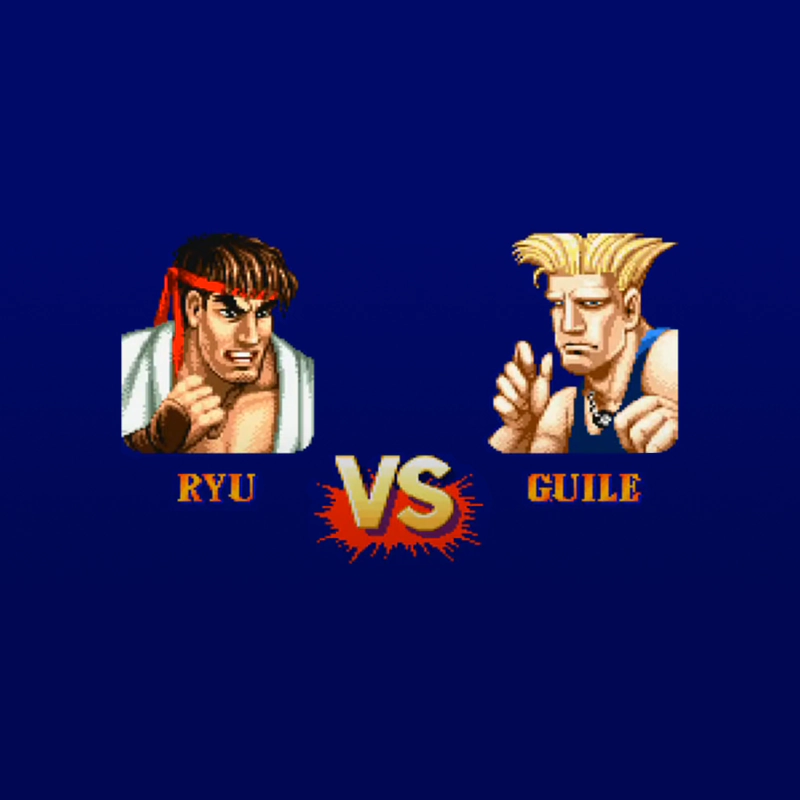
If ever AI should walk the surface of the Earth like proper humans, they need to have sensors to help them map their surroundings, and respond to stimuli.
While the AI field was rather quiet before OpenAI released ChatGPT, researchers have long tried developing both the hardware and software needed for computers to be able to mimic human beings.
And this time, the researchers at Singapore University of Technology and Design (SUTD) have achieved a groundbreaking milestone by harnessing the power of reinforcement learning to train AI.
They showcased this achievement with an AI that can defeat champion Street Fighter players.
The innovative approach used in their research is meant to help reshape movement science, with implications spanning from robotics and autonomous vehicles to collaborative robots and aerial drones, according to the study.
Researchers have previously showcased benchmark of AI development progress using AIs that master various games.
From defeating the world's Go champion to mastering the game of chess through self-play, researchers have also developed AI that can defeat StarCraft II's built-in "AI" in full matches, and another that beats the same game through 200 years worth of training.
One of which even managed to receive the Grandmaster status after outperformed 99.8% of all registered human players.
There is also an AI that masters the classic first-person-shooter game Doom, and another that drives faster than 50,000 human drivers in Gran Turismo Sport, and another that bests the world's best Dota 2 player.
Following those successful projects, the SUTD research team decided to also explore the realm of video games, focusing on Street Fighter, a popular fighting game known for its intricate combat mechanics.
In their quest to create an AI capable of outperforming human players, the team developed a unique movement design software powered by reinforcement learning, a type of machine learning where algorithms learn through experimentation and feedback.
The AI was tasked with learning and refining its movements by competing against built-in AI opponents.
The AI is able to interact in real-time, and respond to decision through the game's system.
The researchers created this SF R2 agent using a previously unreported model-free, natural, deep reinforcement learning algorithm dubbed as the "Decay-based Phase-change memristive character-type Proximal Policy Optimization", or DP-PPO, through an assemblage of hybrid case-type training processes; and an integrated training configuration for time-trial evaluations, as well as competitions with a world's best SF player, is developed.
This approach creates an efficient and effective approach, where the AI can better handle less accurate information to achieve its goals.
This AI exhibited exceptional physical and mental qualities, an accomplishment termed "effective movement design," that is learned with fewer training steps.
In short, according to the research's abstract, , the AI can recapitulate complex multicharacter interactions and, concurrently, generate the millisecond-level control challenges of human athletes.
The result is an AI capable of attacking and dodging enemy attacks, as well as acquiring rapid, effective head-to-head competitive abilities that match the world's best Street Fighter players.
This paves the way for achieving a broadly applicable training scheme, capable of quickly controlling complicated-movement systems in fields where agents should observe unspecified human norms.
"The results were astonishing," said Desmond Loke, associate professor at SUTD and the study's principal investigator.
"Our findings demonstrate that reinforcement learning can do more than just master simple board games. The program excelled in creating more complex movements when trained to address long-standing challenges in movement science."
The implications of this research extend far beyond the realm of gaming. Reinforcement learning can pave the way for advancements in various scientific fields. Associate Prof. Loke emphasized,
"If this method is applied to the right research problems, it could accelerate progress in various scientific fields."
The researchers are optimistic about the future possibilities unlocked by their AI approach. Associate Prof. Loke envisions a world where this technology enables the creation of movements, skills, and actions previously deemed impossible.
"The more effective the technology becomes, the more potential applications it opens up, including the continued progression of competitive tasks that computers can facilitate for the best players, such as in Poker, Starcraft, and Jeopardy," Associate Prof. Loke said. "We may also see high-level realistic competition for training professional players, discovering new tactics, and making video games more interesting."
In conclusion, the research conducted at SUTD represents a significant leap forward in AI, where reinforcement learning allowed AI to master complex tasks beyond traditional board games.